Helping transit operators improve their planning with predictive maintenance
Can data predict the future? This is what predictive maintenance aims to do. As onboard sensors increase the volume of data being collected and artificial intelligence optimizes the use of data, predictive maintenance is becoming an essential counterpart to traditional preventive and curative maintenance. It enables a more agile approach – continuous and in real time – while limiting the need for expensive curative maintenance operations.
In Boston, for example, trains equipped with sensors record vibrations, measure the temperature of trains and detect door malfunctions. This data is centralized and analyzed in real time by predictive maintenance software. AI thus makes it possible to anticipate potential incidents and take action before they occur.
Another example is the Keolis Group’s Citadis project in Lyon. This portal collects all the operating data of the city’s tramways and uploads it to the server when trams return to the depot. This data then helps the operator anticipate breakdowns and accelerate decision-making.
The result? Firstly, optimized service thanks to repairs and replacements of parts being carried out in advance, thus reducing the risk of vehicles breaking down and bottlenecks developing. Secondly, reduced vehicle downtime and more efficient scheduling of maintenance operations for technicians.
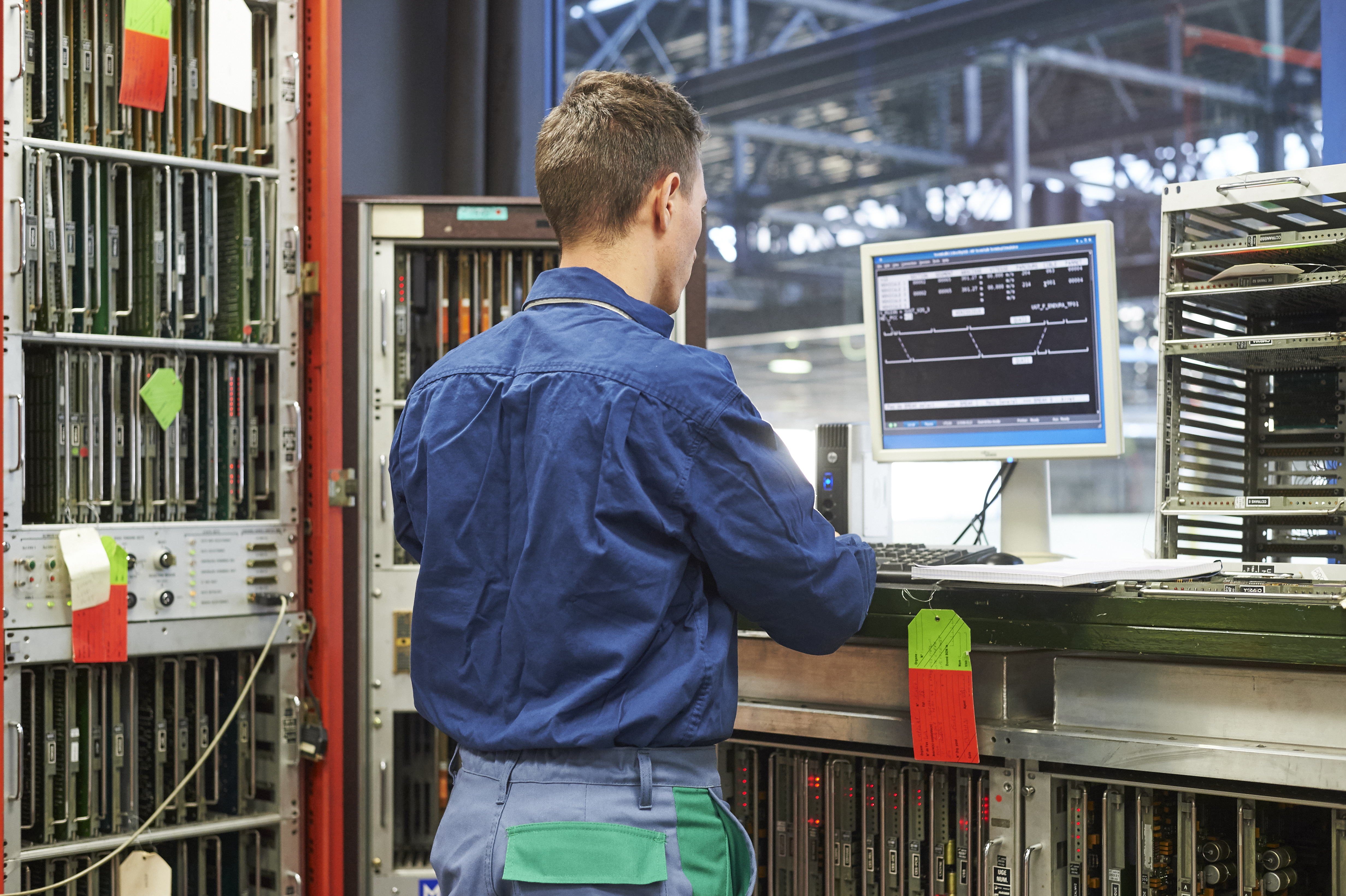